Machine learning models for brain imaging in epilepsy
Practical problems, challenges, and future research directions
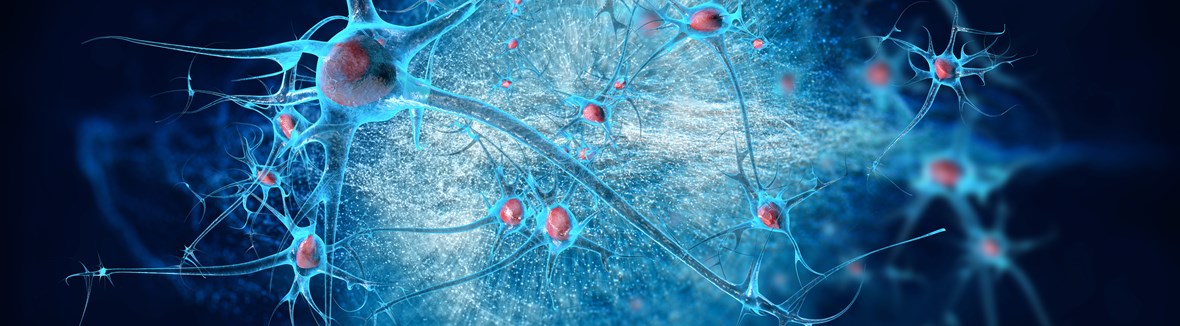
Machine learning is an emerging trend in medicine that encompasses the fields of neurology and epileptology. The advantages of machine learning over conventional methods include accurate, automated, and fast pattern learning. Recent clinical and experimental applications of machine learning for epilepsy include automatic seizure detection from clinical data, pre-surgical planning, prediction of medical and surgical
outcomes, and automated neuroimaging analyses. Neuroimaging is one of the clinically essential exam for epilepsy.
While the main role of neuroimaging in epilepsy is the detection of the focus lesion in drug-resistant epilepsy, there is now promising evidence of the further utility of neuroimaging, such as the of cognitive functions and postsurgical seizure outcomes in epilepsy. The neuroimaging modalities MRI and PET are widely used in
clinical practice and have been thoroughly investigated. Given the rapid development in neuroimaging techniques and machine learning, both of these techniques can be expected to undergo to further progress.
Sone et al. recently reviewed the existing applications of machine learning for brain MRI and PET in epilepsy, and provided further comment on the practical problems, challenges, and future research directions of machine learning in brain imaging for epilepsy.
Distinguishing individuals with epilepsy from healthy controls
At present, machine learning classifiers have achieved over 70–80% accuracy to successfully discriminate between individuals with epilepsy and healthy controls, using T1 images, diffusion MRI, and functional MRI. Studies targeting temporal lobe epilepsy (TLE) have achieved around 90% accuracy, but it has been more difficult to identify idiopathic generalized epilepsy (IGE), with only about 75% accuracy. Despite these successes, the clinical utility of these findings may be limited, since differentiation between individuals with epilepsy and healthy subjects is not a major goal of neuroimaging.
Lateralization of the temporal lobe epilepsy focus
Several machine learning approaches to the lateralization of TLE foci have been employed, using T1-weighted images, diffusion MRI, FLAIR images, 18F-FDG-PET, or a combination of these. The applications of these approaches to cases without visually detectable lesions, i.e., so-called MRI-negative TLE, would be particularly beneficial in clinical settings by providing further clues to the focus beyond the conventional approaches. Although the current accuracy of machine learning lateralization for MRI-negative TLE is not as high as that for MRI-positive cases (> 98%), this approach has achieved >75% accuracy, which would be acceptable in clinical practice.
Machine learning (ML) is an emerging trend in the field of neuroimaging in epilepsy. The advantages of ML over conventional methods include accurate, automated, and fast pattern learning, which can be used to develop and/or optimize clinically useful algorithms for clinical medicine and basic research, but consistent methodologies must yet be developed and validated.
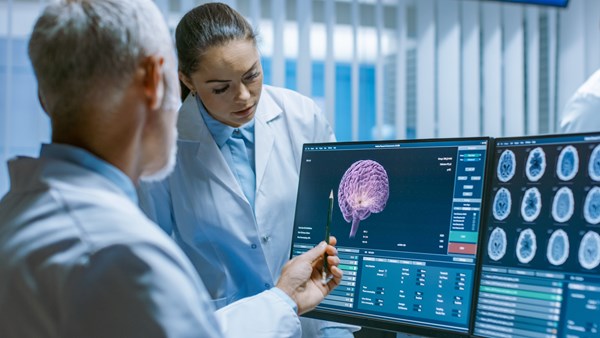
Identification of epileptogenic foci
Accurate localization of the epileptogenic focus is highly relevant for successful epilepsy surgery, which may remediate drug-resistant focal epilepsy. Structural MRI plays a major role in visual detection of focus lesions, and has been widely used in clinical practice. Various applications of machine learning for lesion identification to improve the detection rate or to develop automated algorithms, which would be concordant with the seizure onset zone detected by intracranial EEG. More recent studies tend to use combined data from multimodal imaging, whereas earlier studies used only T1WI.
Prediction of clinical outcomes
A major trend in this section is the prediction of postsurgical seizure freedom, in light of its clinical importance. Most studies report an accuracy of 70–90% for prediction of seizure outcomes after resection surgery. An accuracy of around 85% has been reported for identification of responders to vagus nerve stimulation. In terms of surgery, machine learning methods have also been used to obtain good predictive values for postsurgical functional deficit, lateralization of the language hemisphere, and optimal planning for laser surgery. Cognitive dysfunctions in epilepsy can also be predicted by machine learning methods, as can functional neuroimaging and/or network measurements. Other machine learning applications in this area include prediction of drug responsiveness, acquiring epileptogenicity, and the differentiation of types of autoantibodies.
Brain age prediction
Another trend in the field of neuroimaging and machine learning is regression models, which have often been used for prediction of brain aging. To investigate relationships between brain aging and epilepsy, several groups have employed the regression model technique. In general, an increase in the age of the brain by around 4–10 years has been reported, which is consistent with recent evidence of disease progression or tau deposition in epilepsy. The increased brain age in individuals with epilepsy appears to be associated with longer disease duration, early onset age and/or psychiatric comorbidity.
Problems, challenges, and future directions
Feature extraction is a significant factor in the diversity of research in machine learning. While most studies have used a region-of-interest (ROI) to extract imaging features, the choice of atlases for ROIs varies. Some investigations have used traditional automated anatomical labeling, while a different atlas has been used in other studies. Radiomics is considered as a novel method to extract imaging data, which this might have greater utility than conventional methods.
For more successful clinical applications, consistent methodologies need to be developed and validated. Regarding machine learning algorithms, recent studies have tended to use deep-learning methods such as a convolutional neural network. There is also a clear lack of studies using unsupervised classification. Given the potential of unsupervised clustering to identify hidden patterns in unlabeled data, further studies with unsupervised classification are needed to uncover data-driven information. Lastly, new software is being developed that uses a single EEG recording of brain activity to identify epilepsy, with a similar sensitivity to that of clinicians who conduct numerous tests over many months.
- Sone D, Beheshti I. Clinical Application of Machine Learning Models for Brain Imaging in Epilepsy: A Review. Front Neurosci. 2021;15:684825.
- Bender E. Accelerating the diagnosis of epilepsy with computer modelling. Nature. 2021 Jun 24. doi: 10.1038/d41586-021-01666-9.
SW13947P, November 2022